技术专栏
MMdetect的使用
MMdetect 使打开配置文件用说明
" class="reference-link">数据集的格式为COCO格式
coco 文件脚本
XML to json
import os
import json
import random
import xml.etree.ElementTree as ET
import glob
def get(root, name):
vars = root.findall(name)
return vars
def get_and_check(root, name, length):
vars = root.findall(name)
if len(vars) == 0:
raise ValueError("Can not find %s in %s." % (name, root.tag))
if length > 0 and len(vars) != length:
raise ValueError(
"The size of %s is supposed to be %d, but is %d."
% (name, length, len(vars))
)
if length == 1:
vars = vars[0]
return vars
def get_categories(xml_files):
classes_names = []
for xml_file in xml_files:
tree = ET.parse(xml_file)
root = tree.getroot()
for member in root.findall("object"):
classes_names.append(member[0].text)
classes_names = list(set(classes_names))
classes_names.sort()
return {name: i for i, name in enumerate(classes_names)}
def generate_label_file(xml_files, json_file):
json_dict = {"images": [], "type": "instances", "annotations": [], "categories": []}
if classes:
categories = classes
else:
categories = get_categories(xml_files)
bnd_id = 1
image_id = 1
for xml_file in xml_files:
tree = ET.parse(xml_file)
root = tree.getroot()
path = get(root, "path")
if len(path) == 1:
filename = os.path.basename(path[0].text)
elif len(path) == 0:
filename = get_and_check(root, "filename", 1).text
else:
raise ValueError("%d paths found in %s" % (len(path), xml_file))
size = get_and_check(root, "size", 1)
width = int(get_and_check(size, "width", 1).text)
height = int(get_and_check(size, "height", 1).text)
image = {
"file_name": filename,
"height": height,
"width": width,
"id": image_id,
}
json_dict["images"].append(image)
for obj in get(root, "object"):
category = get_and_check(obj, "name", 1).text
if category not in categories:
print(f'{category} not in classes')
continue
category_id = categories[category]
bndbox = get_and_check(obj, "bndbox", 1)
xmin = int(get_and_check(bndbox, "xmin", 1).text) - 1
ymin = int(get_and_check(bndbox, "ymin", 1).text) - 1
xmax = int(get_and_check(bndbox, "xmax", 1).text)
ymax = int(get_and_check(bndbox, "ymax", 1).text)
assert xmax > xmin
assert ymax > ymin
o_width = abs(xmax - xmin)
o_height = abs(ymax - ymin)
ann = {
"area": o_width * o_height,
"iscrowd": 0,
"image_id": image_id,
"bbox": [xmin, ymin, o_width, o_height],
"category_id": category_id,
"id": bnd_id,
"ignore": 0,
"segmentation": [],
}
json_dict["annotations"].append(ann)
bnd_id += 1
image_id += 1
for cate, cid in categories.items():
cat = {"supercategory": "none", "id": cid, "name": cate}
json_dict["categories"].append(cat)
os.makedirs(os.path.dirname(json_file), exist_ok=True)
json_fp = open(json_file, "w")
json_str = json.dumps(json_dict)
json_fp.write(json_str)
json_fp.close()
if __name__ == "__main__":
# xml文件地址
xml_path = "/home/censoft/2tbdataset/ysc_dataset/xianyu_guaika/Annotations"
xml_files = glob.glob(os.path.join(xml_path, "*.xml"))
# 定义类别字典
# ["1c" ,"2c" ,"3c", "1" ,"2" ,"xzw" ,"gd" ,"hg"]
classes = {'1c': 0, '2c': 1,'3c': 2, '1': 3,'2': 4, 'xzw': 5,'gd': 6, 'hg': 7} # classes为空时,自动遍历读取xml文件中的所有类别并生成字典
# 生成的json文件保存地址及文件名
json_file = "/home/censoft/2tbdataset/ysc_dataset/xianyu_guaika/Annotations/json/train.json"
# 执行转换
generate_label_file(xml_files, json_file)
jsontococo
import os
import json
import numpy as np
import glob
import shutil
import cv2
from sklearn.model_selection import train_test_split
# 1 将数据集改成coco
# 2 mmdet-->dataset-->coco 中的类别更改
# 3 mmdet-->core-->evaluation-->class_names.py 中的coco_classes 类的返回值
# 4 tools/work_dirs/deformable_detr_r50_16x2_50e_coco/my_deformable_detr_r50_16x2_50e_coco.py 将 num_classes=2, 改成自己的
# 5 复制D:\\python\\ai\\mmdetection-master\\configs\\deformable_detr\\deformable_detr_r50_16x2_50e_coco.py 路径
# 5.1 去到 tools/train.py 修改运行配置运行 会生成一个文件在配置在 tools/work_dirs/deformable_detr_r50_16x2_50e_coco/my_deformable_detr_r50_16x2_50e_coco.py
# 5.1 将该配置复制到 configs/deformable_detr 使用我们自己的配置 不要动原来的配置文件
np.random.seed(41)
classname_to_id = {'1c': 0, '2c': 1,'3c': 2, '1': 3,'2': 4, 'xzw': 5,'gd': 6, 'hg': 7} # classes为空时,自动遍历读取xml文件中的所有类别并生成字典
class Lableme2CoCo:
def __init__(self):
self.images = []
self.annotations = []
self.categories = []
self.img_id = 0
self.ann_id = 0
def save_coco_json(self, instance, save_path):
json.dump(instance, open(save_path, 'w', encoding='utf-8'), ensure_ascii=False, indent=1) # indent=2 更加美观显示
# 由json文件构建COCO
def to_coco(self, json_path_list):
self._init_categories()
for json_path in json_path_list:
obj = self.read_jsonfile(json_path)
self.images.append(self._image(obj, json_path))
shapes = obj['shapes']
for shape in shapes:
annotation = self._annotation(shape)
self.annotations.append(annotation)
self.ann_id += 1
self.img_id += 1
instance = {}
instance['info'] = 'spytensor created'
instance['license'] = ['license']
instance['images'] = self.images
instance['annotations'] = self.annotations
instance['categories'] = self.categories
return instance
# 构建类别
def _init_categories(self):
for k, v in classname_to_id.items():
category = {}
category['id'] = v
category['name'] = k
self.categories.append(category)
# 构建COCO的image字段
def _image(self, obj, path):
image = {}
# from labelme import utils
# img_x = utils.img_b64_to_arr(obj['imageData'])
img_x=cv2.imread(obj['imagePath'])
h, w = img_x.shape[:-1]
image['height'] = h
image['width'] = w
image['id'] = self.img_id
image['file_name'] = os.path.basename(path).replace(".json", ".jpg")
return image
# 构建COCO的annotation字段
def _annotation(self, shape):
# print('shape', shape)
label = shape['label']
points = shape['points']
annotation = {}
annotation['id'] = self.ann_id
annotation['image_id'] = self.img_id
annotation['category_id'] = int(classname_to_id[label])
annotation['segmentation'] = [np.asarray(points).flatten().tolist()]
annotation['bbox'] = self._get_box(points)
annotation['iscrowd'] = 0
annotation['area'] = 1.0
return annotation
# 读取json文件,返回一个json对象
def read_jsonfile(self, path):
with open(path, "r", encoding='utf-8') as f:
return json.load(f)
# COCO的格式: [x1,y1,w,h] 对应COCO的bbox格式
def _get_box(self, points):
min_x = min_y = np.inf
max_x = max_y = 0
for x, y in points:
min_x = min(min_x, x)
min_y = min(min_y, y)
max_x = max(max_x, x)
max_y = max(max_y, y)
return [min_x, min_y, max_x - min_x, max_y - min_y]
#训练过程中,如果遇到Index put requires the source and destination dtypes match, got Long for the destination and Int for the source
#参考:https://github.com/open-mmlab/mmdetection/issues/6706
if __name__ == '__main__':
labelme_path = "/home/censoft/2tbdataset/ysc_dataset/xianyu_guaika/json"
saved_coco_path = "/home/censoft/2tbdataset/ysc_dataset/xianyu_guaika/"
images_path="/home/censoft/2tbdataset/ysc_dataset/xianyu_guaika/JPEGImages/"
print('reading...')
# 创建文件
if not os.path.exists("%scoco/annotations/" % saved_coco_path):
os.makedirs("%scoco/annotations/" % saved_coco_path)
if not os.path.exists("%scoco/images/train2017/" % saved_coco_path):
os.makedirs("%scoco/images/train2017" % saved_coco_path)
if not os.path.exists("%scoco/images/val2017/" % saved_coco_path):
os.makedirs("%scoco/images/val2017" % saved_coco_path)
# 获取images目录下所有的joson文件列表
print(labelme_path + "/*.json")
json_list_path = glob.glob(labelme_path + "/*.json")
print('json_list_path: ', len(json_list_path))
# 数据划分,这里没有区分val2017和tran2017目录,所有图片都放在images目录下
train_path, val_path = train_test_split(json_list_path, test_size=0.1, train_size=0.9)
print("train_n:", len(train_path), 'val_n:', len(val_path))
# 把训练集转化为COCO的json格式
l2c_train = Lableme2CoCo()
train_instance = l2c_train.to_coco(train_path)
l2c_train.save_coco_json(train_instance, '%scoco/annotations/instances_train2017.json' % saved_coco_path)
for file in train_path:
# shutil.copy(file.replace("json", "jpg"), "%scoco/images/train2017/" % saved_coco_path)
img_name = file.split('/')[-1][:-4]
temp_img = cv2.imread(images_path+img_name+'jpg')
try:
cv2.imwrite("{}coco/images/train2017/{}".format(saved_coco_path, img_name.split('\\')[-1]+'jpg'), temp_img)
except Exception as e:
print(e)
print('Wrong Image1:', img_name )
continue
print(img_name + '-->', img_name.replace('png', 'jpg'))
for file in val_path:
# shutil.copy(file.replace("json", "jpg"), "%scoco/images/val2017/" % saved_coco_path)
# img_name = file.replace('json', 'jpg')
img_name = file.split('/')[-1][:-4]
temp_img = cv2.imread(images_path+img_name+'jpg')
try:
cv2.imwrite("{}coco/images/val2017/{}".format(saved_coco_path, img_name.split('\\')[-1]+'jpg'), temp_img)
except Exception as e:
print(e)
print('Wrong Image2:', img_name)
continue
print(img_name + '-->', img_name.replace('png', 'jpg'))
# 把验证集转化为COCO的json格式
l2c_val = Lableme2CoCo()
val_instance = l2c_val.to_coco(val_path)
l2c_val.save_coco_json(val_instance, '%scoco/annotations/instances_val2017.json' % saved_coco_path)
修改文件
第一处: mmdet/evaluation/functional/class_names.py 代码下的 def coco_classes() 的 return 内容改为自己数据集的类别;
第二处:mmdet/datasets/coco.py 代码下的 class CocoDataset(CustomDataset) 的 CLASSES 改为自己数据集的类别;
注意:修改两处后,一定要在根目录下,输入命令:
python setup.py install build
重新编译代码,要不然类别会没有载入,还是原coco类别,训练异常。
训练
python tools/train.py configs/cascade_rcnn/cascade_rcnn_r50_fpn_1x_coco.py --work-dir work_dirs
执行该命令以后会在work_dirs生成一个新的配置文件
打开配置文件 my_faster-rcnn_r50_fpn_1x_coco.py
修改data_root
路径和训练集、验证集、测试集的图片和标签路径,如下图:
正式训练
!!!看清楚路径!使用的是更改过的配置文件训练!!!
python tools/train.py work_dirs/my_cascade_rcnn_r50_fpn_1x_coco.py
检测
将检测图片转成json
python tools/dataset_converters/images2coco.py /home/censoft/2tbdataset/ysc_dataset/xianyu_guaika/ceshi [图片地址] /home/censoft/2tbdataset/ysc_dataset/xianyu_guaika/labels.txt 【标签文件】 detect.json 【结果】
然后将json 文件换成detect.json
MMYolo2的使用
说明需要安装 mmdetect
注意:训练时需要更改 mmdetect 里面的类别,修改步骤如上面 修改文件
其他一致
声明:本文内容由易百纳平台入驻作者撰写,文章观点仅代表作者本人,不代表易百纳立场。如有内容侵权或者其他问题,请联系本站进行删除。
红包
点赞
收藏
评论
打赏
- 分享
- 举报
评论
0个
手气红包
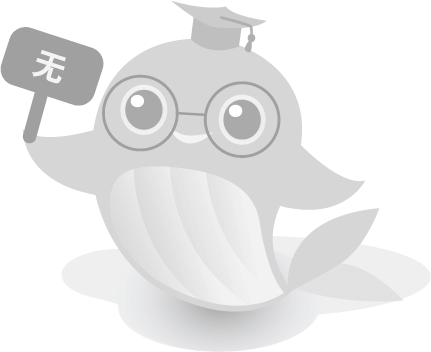
相关专栏
-
浏览量:1746次2020-08-14 18:41:11
-
浏览量:2592次2020-08-04 15:11:02
-
浏览量:1477次2020-09-09 19:14:23
-
浏览量:6781次2018-01-22 14:23:15
-
浏览量:6481次2022-01-07 09:00:13
-
浏览量:4308次2020-08-18 19:31:22
-
浏览量:2942次2019-12-05 17:38:34
-
浏览量:10319次2020-08-20 11:12:38
-
浏览量:9303次2020-08-18 21:11:17
-
浏览量:2752次2017-11-22 11:51:03
-
浏览量:1222次2023-07-27 10:34:40
-
浏览量:1459次2020-03-23 13:40:43
-
浏览量:5602次2021-02-09 14:27:57
-
浏览量:6806次2020-12-22 09:37:22
-
浏览量:3674次2020-08-18 15:39:19
-
浏览量:1314次2023-09-20 17:34:18
-
浏览量:2895次2020-05-14 17:30:06
-
浏览量:705次2023-12-21 10:38:07
-
浏览量:1547次2024-01-12 15:38:50
切换马甲
上一页
下一页
打赏用户
共 0 位
我要创作
分享技术经验,可获取创作收益
热门专栏
- 【深度学习】卷积神经网络-图片分类案例(pytorch实现)
- 【深度学习】基于Torch的Python开源机器学习库PyTorch概述
- 卷积神经网络resent网络实践
- 基于深度学习框架的离网DOA估计
- 海思平台深度学习算法仿真使用RuyiStudio生成wk文件
- Pytorch版本YOLOv3模型转Darknet weights模型然后转caffemodel再转wk模型在nnie上面推理
- 机器学习中的静态图与动态图
- 基于tensorflow深度学习的猫狗分类识别
- pytorch 导出 onnx 模型 & 用onnxruntime 推理图片
- 深度学习基础:使用TensorFlow 了解overfitting 与underfittin
置顶时间设置
结束时间
删除原因
-
广告/SPAM
-
恶意灌水
-
违规内容
-
文不对题
-
重复发帖
打赏作者
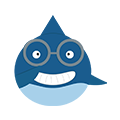
shui
您的支持将鼓励我继续创作!
打赏金额:
¥1

¥5

¥10

¥50

¥100

支付方式:

举报反馈
举报类型
- 内容涉黄/赌/毒
- 内容侵权/抄袭
- 政治相关
- 涉嫌广告
- 侮辱谩骂
- 其他
详细说明
审核成功
发布时间设置
发布时间:
请选择发布时间设置
是否关联周任务-专栏模块
审核失败
失败原因
请选择失败原因
备注
请输入备注