Tensorflow Lite 入门——模型的训练和转换
之前的文章中介绍的使用 Tensorflow 训练的模型要么只能运行在 PC 端,要么需要云端的支持。随着智能手机和物联网设备的普及,能够在智能手机甚至嵌入式设备直接运行的模型需求就越来越高。这篇文章就开始介绍 Tensorflow Lite, 这个能够运行在智能手机和 嵌入式设备的开源深度学习框架。
通常,我们会在 PC 或者云端建立模型,并对模型进行训练,然后将模型转换成 Tensorflow Lite 的格式,并最终部署到终端设备上,这篇文章我们就用 Fashion MNIST 的数据集,建立并训练模型,并采用模拟器的方式部署到终端设备上进行测试。
1. 数据的加载和训练
这个部分的内容与之前Tensorflow 2.0 快速入门内容重复,在这里就不过多赘述了。但是值得注意的是,之前的文章我们都是使用的 keras 的数据集,其数据格式是 numpy。Fasion MINST 是从 tensorflow_datasets
中直接加载的。将数据集分为 80% 训练集,10% 测试集和10%验证集。tfds的详细使用说明请参考官方文档。
https://www.tensorflow.org/datasets/api_docs/python/tfds/load
import tensorflow_datasets as tfds
splits, info = tfds.load('fashion_mnist', with_info=True, as_supervised=True,
split=['train[:80%]', 'train[80%:90%]', 'train[90%:]'])
(train_examples, validation_examples, test_examples) = splits
num_examples = info.splits['train'].num_examples
num_classes = info.features['label'].num_classes
- 1
- 2
- 3
- 4
- 5
- 6
- 7
- 8
- 9
数据经过预处理之后,我们使用 Keras 的 API 快速搭建了一个五层的 CNN 神经网络并使用 model.fit
对模型进行了训练。
2. 模型的保存与转换
训练好的模型这里使用了 tf.save_model.save() 将模型保存在了指定目录。
export_dir = 'saved_model/1'
# Use the tf.saved_model API to export the SavedModel
tf.saved_model.save(model, export_dir)
- 1
- 2
- 3
- 4
本文的重点是模型转换,在Tensorflow Lite 中,使用 TFLiteCoverter
可以轻松将模型转换成 Tensorflow Lite 的模型。
# Use the TFLiteConverter SavedModel API to initialize the converter
converter = tf.lite.TFLiteConverter.from_saved_model(saved_model_dir=export_dir)
# Invoke the converter to finally generate the TFLite model
tflite_model = converter.convert()
# Save the model file as 'model.tflite'
tflite_model_file = 'model.tflite'
with open(tflite_model_file, "wb") as f:
f.write(tflite_model)
- 1
- 2
- 3
- 4
- 5
- 6
- 7
- 8
- 9
- 10
3. 模型的优化
converter 在默认的情况下是将模型权重从32位浮点数转换成8位整数从而大大减小模型的大小。
converter.optimizations = [tf.lite.Optimize.DEFAULT]
- 1
我们也可以将模型手动调整为16位浮点数
converter.optimizations = [tf.lite.Optimize.DEFAULT]
converter.target_spec.supported_types = [tf.float16]
- 1
- 2
当然除了 default 模式,官方也提供了精度优先和大小优先的优化模式,详细内容参考官方文档
https://www.tensorflow.org/lite/performance/post_training_quantization
tf.lite.Optimize.OPTIMIZE_FOR_LATENCY
tf.lite.Optimize.OPTIMIZE_FOR_SIZE
- 1
- 2
4. 模型测试
到这里,实际上我们就可以将转换成 Tensorflow Lite 的模型部署到设备上进行测试了,但是此时,我们并不知道模型的性能如何,Tensorflow Lite 提供了模拟器,我们可以轻松部署在模拟器上对转换后的模型进行测试。
测试模型分为三步
第一步:加载 TFLite 模型,部署tensor
第二步:获取 input 和 output index
第三步:加载数据并获取结果
# Step 1: Load TFLite model and allocate tensors.
interpreter = tf.lite.Interpreter(model_content=tflite_model)
interpreter.allocate_tensors()
# Step 2: Get input and output tensors index
input_index = interpreter.get_input_details()[0]["index"]
output_index = interpreter.get_output_details()[0]["index"]
# Step 3: Get results
interpreter.set_tensor(input_index, img)
interpreter.invoke()
predictions = interpreter.get_tensor(output_index)
- 1
- 2
- 3
- 4
- 5
- 6
- 7
- 8
- 9
- 10
- 11
- 12
5. 总结
Tensorflow Lite 在PC 或者云端的训练和测试可以分为三个步骤:1. 数据加载,模型搭建与的训练。2. 模型的保存与转换。3.模型的测试。
经过这三个步骤之后,我们就可以将转换后的模型部署在终端设备上了,Tensorflow Lite 不仅可以支持 Android 和 iOS 的智能手机也支持raspberry pi 智能手环这样的嵌入式设备,如果有机会将在后面的文章中给大家介绍。
- 分享
- 举报
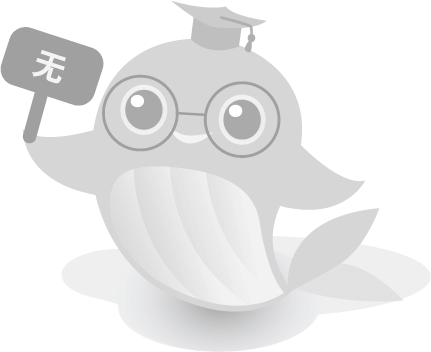
-
2024-12-10 11:25:33
-
浏览量:6023次2021-07-30 10:33:41
-
浏览量:253次2025-02-26 21:18:20
-
2024-12-10 11:13:53
-
2023-11-23 14:00:42
-
浏览量:1397次2019-12-09 10:34:06
-
2024-12-10 10:52:33
-
浏览量:1773次2024-02-23 17:41:04
-
浏览量:11592次2020-12-16 17:38:28
-
浏览量:1792次2020-01-04 10:19:25
-
2024-12-10 10:34:18
-
浏览量:5085次2021-04-21 17:06:33
-
浏览量:8305次2020-12-13 17:30:07
-
浏览量:2717次2024-01-05 14:11:13
-
浏览量:5712次2021-05-17 16:52:58
-
浏览量:5829次2021-07-15 10:44:33
-
浏览量:18511次2021-07-15 10:45:21
-
浏览量:2596次2024-02-02 18:15:06
-
浏览量:3144次2020-12-12 11:07:57
-
广告/SPAM
-
恶意灌水
-
违规内容
-
文不对题
-
重复发帖

一休摸鱼






举报类型
- 内容涉黄/赌/毒
- 内容侵权/抄袭
- 政治相关
- 涉嫌广告
- 侮辱谩骂
- 其他
详细说明