【深度学习】实战Kaggle竞赛之线性模型解决波士顿房价预测问题
【深度学习】实战Kaggle竞赛之线性模型解决波士顿房价预测问题
文章目录
1 概述
1.1 Competition Description
1.2 Practice Skills
2 数据处理
3 训练和测试
4 提交
1 概述
1.1 Competition Description
Ask a home buyer to describe their dream house, and they probably won't begin with the height of the basement ceiling or the proximity to an east-west railroad. But this playground competition's dataset proves that much more influences price negotiations than the number of bedrooms or a white-picket fence.
With 79 explanatory variables describing (almost) every aspect of residential homes in Ames, Iowa, this competition challenges you to predict the final price of each home.
1.2 Practice Skills
Creative feature engineering
Advanced regression techniques like random forest and gradient boosting
2 数据处理
注意,竞赛数据分为训练集和测试集。每条记录都包括房屋的属性值和属性,如街道类型、施工年份、屋顶类型、地下室状况等。这些特征由各种数据类型组成。例如,建筑年份由整数表示,屋顶类型由离散类别表示,其他特征由浮点数表示。这就是现实让事情变得复杂的地方:例如,一些数据完全丢失了,缺失值被简单地标记为“NA”。每套房子的价格只出现在训练集中(毕竟这是一场比赛)。我们将希望划分训练集以创建验证集,但是在将预测结果上传到Kaggle之后,我们只能在官方测试集中评估我们的模型。在 :numref:fig_house_pricing 中,"Data"选项卡有下载数据的链接。
开始之前,我们将[使用pandas读入并处理数据],因此,在继续操作之前,您需要确保已安装pandas。幸运的是,如果你正在用Jupyter阅读该书,你可以在不离开笔记本的情况下安装pandas。
# 如果pandas没有被安装,请取消下一句的注释。
# !pip install pandas
%matplotlib inline
import numpy as np
import pandas as pd
import torch
from torch import nn
from d2l import torch as d2l
DATA_HUB['kaggle_house_train'] = ( #@save
DATA_URL + 'kaggle_house_pred_train.csv',
'585e9cc93e70b39160e7921475f9bcd7d31219ce')
DATA_HUB['kaggle_house_test'] = ( #@save
DATA_URL + 'kaggle_house_pred_test.csv',
'fa19780a7b011d9b009e8bff8e99922a8ee2eb90')
我们使用pandas分别加载包含训练数据和测试数据的两个CSV文件。
train_data = pd.read_csv(download('kaggle_house_train'))
test_data = pd.read_csv(download('kaggle_house_test'))
print(train_data.shape)
print(test_data.shape)
让我们看看[前四个和最后两个特征,以及相应标签](房价)。
print(train_data.iloc[0:4, [0, 1, 2, 3, -3, -2, -1]])
我们可以看到,(在每个样本中,第一个特征是ID,)这有助于模型识别每个训练样本。虽然这很方便,但它不携带任何用于预测的信息。因此,在将数据提供给模型之前,(我们将其从数据集中删除)。
all_features = pd.concat((train_data.iloc[:, 1:-1], test_data.iloc[:, 1:]))
如上所述,我们有各种各样的数据类型。在开始建模之前,我们需要对数据进行预处理。让我们从数字特征开始。首先,我们应用启发式方法,[将所有缺失的值替换为相应特征的平均值。]然后,为了将所有特征放在一个共同的尺度上,我们(通过将特征重新缩放到零均值和单位方差来标准化数据):
$$x \leftarrow \frac{x - \mu}{\sigma}.$$
要验证这确实转换了我们的特征(变量),使特征具有零均值和单位方差,即 $E[\frac{x-\mu}{\sigma}] = \frac{\mu - \mu}{\sigma} = 0$和$E[(x-\mu)^2] = (\sigma^2 + \mu^2) - 2\mu^2+\mu^2 = \sigma^2$。直观地说,我们标准化数据有两个原因。首先,它方便优化。其次,因为我们不知道哪些特征是相关的,所以我们不想让惩罚分配给一个特征的系数比分配给其他任何特征的系数更大。
numeric_features = all_features.dtypes[all_features.dtypes != 'object'].index
all_features[numeric_features] = all_features[numeric_features].apply(
lambda x: (x - x.mean()) / (x.std()))
# 在标准化数据之后,所有数据都意味着消失,因此我们可以将缺失值设置为0
all_features[numeric_features] = all_features[numeric_features].fillna(0)
接下来,我们[处理离散值。]这包括诸如“MSZoning”之类的特征。(我们用一次独热编码替换它们),方法与前面将多类别标签转换为向量的方式相同。例如,“MSZoning”包含值“RL”和“Rm”。将创建两个新的指示器特征“MSZoning_RL”和“MSZoning_RM”,其值为0或1。根据独热编码,如果“MSZoning”的原始值为“RL”,则:“MSZoning_RL”为1,“MSZoning_RM”为0。pandas
软件包会自动为我们实现这一点。
# `Dummy_na=True` 将“na”(缺失值)视为有效的特征值,并为其创建指示符特征。
all_features = pd.get_dummies(all_features, dummy_na=True)
all_features.shape
此转换会将特征的数量从79个增加到331个。最后,通过values属性,我们可以[从pandas格式中提取NumPy格式,并将其转换为张量表示]用于训练。
n_train = train_data.shape[0]
train_features = torch.tensor(all_features[:n_train].values, dtype=torch.float32)
test_features = torch.tensor(all_features[n_train:].values, dtype=torch.float32)
train_labels = torch.tensor(
train_data.SalePrice.values.reshape(-1, 1), dtype=torch.float32)
3 训练和测试
loss = nn.MSELoss()
in_features = train_features.shape[1]
def get_net():
net = nn.Sequential(nn.Linear(in_features,1))
return net
对于房价,就像股票价格一样,我们关心的是相对数量,而不是绝对数量。因此,[我们更关心相对误差$\frac{y - \hat{y}}{y}$,]而不是绝对误差$y - \hat{y}$。例如,如果我们在俄亥俄州农村地区估计一栋房子的价格时,我们的预测偏差了10万美元,在那里一栋典型的房子的价值是12.5万美元,那么我们可能做得很糟糕。另一方面,如果我们在加州豪宅区的预测出现了这个数字的偏差,这可能是一个惊人的准确预测(在那里,房价均值超过400万美元)。
(解决这个问题的一种方法是用价格预测的对数来衡量差异)。事实上,这也是比赛中官方用来评价提交质量的误差指标。即将 $\delta$ for $|\log y - \log \hat{y}| \leq \delta$转换为$e^{-\delta} \leq \frac{\hat{y}}{y} \leq e^\delta$。这使得预测价格的对数与真实标签价格的对数之间出现以下均方根误差:
$$\sqrt{\frac{1}{n}\sum_{i=1}^n\left(\log y_i -\log \hat{y}_i\right)^2}.$$
def log_rmse(net, features, labels):
# 为了在取对数时进一步稳定该值,将小于1的值设置为1
clipped_preds = torch.clamp(net(features), 1, float('inf'))
rmse = torch.sqrt(loss(torch.log(clipped_preds),
torch.log(labels)))
return rmse.item()
下面的代码将权重衰减自动加入到优化器中,是为了解决过拟合问题,当然,定义优化器自然要传入学习率。
在num_epoch内进行迭代,在一个train_iter内进行迭代,双层循环,其实效率并不高,但也没有其他的实现手段。首先optimizer.zero_grad()
梯度清0,这句话也可以放到其他位置哦,l = loss(net(X), y)
前向传播计算误差,l.backward()
反向传播,计算梯度存储到计算图,optimizer.step()
调用优化器进行参数更新。
整体过程就是这样哦,要牢记于心!!!!!!!!。
def train(net, train_features, train_labels, test_features, test_labels,
num_epochs, learning_rate, weight_decay, batch_size):
train_ls, test_ls = [], []
train_iter = d2l.load_array((train_features, train_labels), batch_size)
# 这里使用的是Adam优化算法
optimizer = torch.optim.Adam(net.parameters(),
lr = learning_rate,
weight_decay = weight_decay)
for epoch in range(num_epochs):
for X, y in train_iter:
optimizer.zero_grad()
l = loss(net(X), y)
l.backward()
optimizer.step()
train_ls.append(log_rmse(net, train_features, train_labels))
if test_labels is not None:
test_ls.append(log_rmse(net, test_features, test_labels))
return train_ls, test_ls
[K折交叉验证]。这有助于模型选择和超参数调整。我们首先需要一个函数,在$K$折交叉验证过程中返回第$i$折的数据。它选择第$i$个切片作为验证数据,其余部分作为训练数据。注意,这并不是处理数据的最有效方法,如果我们的数据集大得多,我们肯定会做一些更聪明的改变。但是这种改变所增加的复杂性可能会使代码看起来更乱。在这里可以忽略这些改变,因为我们的问题很简单。
def get_k_fold_data(k, i, X, y):
assert k > 1
fold_size = X.shape[0] // k
X_train, y_train = None, None
for j in range(k):
idx = slice(j * fold_size, (j + 1) * fold_size)
X_part, y_part = X[idx, :], y[idx]
if j == i:
X_valid, y_valid = X_part, y_part
elif X_train is None:
X_train, y_train = X_part, y_part
else:
X_train = torch.cat([X_train, X_part], 0)
y_train = torch.cat([y_train, y_part], 0)
return X_train, y_train, X_valid, y_valid
当我们在 𝐾 折交叉验证中训练 𝐾 次后,[返回训练和验证误差的平均值]。
def k_fold(k, X_train, y_train, num_epochs, learning_rate, weight_decay,
batch_size):
train_l_sum, valid_l_sum = 0, 0
for i in range(k):
data = get_k_fold_data(k, i, X_train, y_train)
net = get_net()
train_ls, valid_ls = train(net, *data, num_epochs, learning_rate,
weight_decay, batch_size)
train_l_sum += train_ls[-1]
valid_l_sum += valid_ls[-1]
if i == 0:
d2l.plot(list(range(1, num_epochs + 1)), [train_ls, valid_ls],
xlabel='epoch', ylabel='rmse', xlim=[1, num_epochs],
legend=['train', 'valid'], yscale='log')
print(f'fold {i + 1}, train log rmse {float(train_ls[-1]):f}, '
f'valid log rmse {float(valid_ls[-1]):f}')
return train_l_sum / k, valid_l_sum / k
在本例中,我们选择了一组未调优的超参数,并将其留给读者来改进模型。找到一个好的选择可能需要时间,这取决于一个人优化了多少变量。有了足够大的数据集和合理设置的超参数, 𝐾 折交叉验证往往对多次测试具有相当的适应性。然而,如果我们尝试了不合理的大量选项,我们可能会发现验证效果不再代表真正的误差。
k, num_epochs, lr, weight_decay, batch_size = 5, 100, 5, 0, 64
train_l, valid_l = k_fold(k, train_features, train_labels, num_epochs, lr,
weight_decay, batch_size)
print(f'{k}-折验证: 平均训练log rmse: {float(train_l):f}, '
f'平均验证log rmse: {float(valid_l):f}')
请注意,有时一组超参数的训练误差可能非常低,但 𝐾 折交叉验证的误差要高得多。这表明我们过拟合了。在整个训练过程中,你将希望监控训练误差和验证误差这两个数字。较少的过拟合可能表明现有数据可以支撑一个更强大的模型。较大的过拟合可能意味着我们可以通过正则化技术来获益。
既然我们知道应该选择什么样的超参数,我们不妨使用所有数据对其进行训练(而不是仅使用交叉验证中使用的 1−1/𝐾 的数据)。然后,我们通过这种方式获得的模型可以应用于测试集。将预测保存在CSV文件中可以简化将结果上传到Kaggle的过程。
def train_and_pred(train_features, test_feature, train_labels, test_data,
num_epochs, lr, weight_decay, batch_size):
net = get_net()
train_ls, _ = train(net, train_features, train_labels, None, None,
num_epochs, lr, weight_decay, batch_size)
d2l.plot(np.arange(1, num_epochs + 1), [train_ls], xlabel='epoch',
ylabel='log rmse', xlim=[1, num_epochs], yscale='log')
print(f'train log rmse {float(train_ls[-1]):f}')
# 将网络应用于测试集。
preds = net(test_features).detach().numpy()
# 将其重新格式化以导出到Kaggle
test_data['SalePrice'] = pd.Series(preds.reshape(1, -1)[0])
submission = pd.concat([test_data['Id'], test_data['SalePrice']], axis=1)
submission.to_csv('submission.csv', index=False)
preds = net(test_features)
这句话用于预测哦。
train_and_pred(train_features, test_features, train_labels, test_data,
num_epochs, lr, weight_decay, batch_size)
train log rmse 0.162781
4 提交
在这个例子中,不需要提交模型的权重等等,你只需要提交结果文件即可,在下面的网站中提交哦。
https://www.kaggle.com/c/house-prices-advanced-regression-techniques/submissions
就到这啦。
- 分享
- 举报
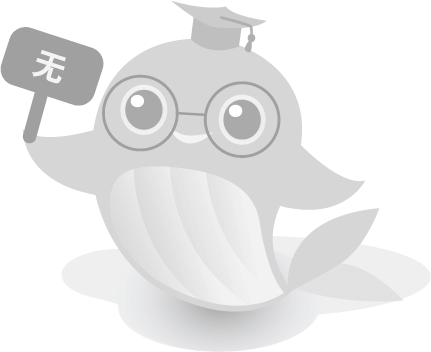
-
浏览量:10854次2021-02-21 21:57:48
-
浏览量:5856次2021-04-14 16:24:29
-
浏览量:1685次2018-02-17 00:18:09
-
浏览量:197次2023-08-15 22:50:27
-
浏览量:1438次2019-07-05 11:07:47
-
浏览量:3674次2018-02-27 19:15:34
-
浏览量:1992次2018-05-14 22:49:29
-
浏览量:5212次2021-02-21 22:45:39
-
浏览量:1420次2018-05-17 15:54:14
-
浏览量:6166次2021-07-26 11:27:40
-
浏览量:7055次2021-07-26 11:30:25
-
浏览量:5024次2021-04-21 17:06:33
-
浏览量:1666次2018-10-16 21:50:29
-
浏览量:1273次2018-06-22 22:05:05
-
浏览量:1698次2019-11-15 08:52:32
-
浏览量:2324次2020-04-24 10:58:46
-
浏览量:1052次2023-03-02 13:55:57
-
浏览量:6109次2021-08-03 11:36:37
-
浏览量:6072次2021-08-03 11:36:18
-
广告/SPAM
-
恶意灌水
-
违规内容
-
文不对题
-
重复发帖
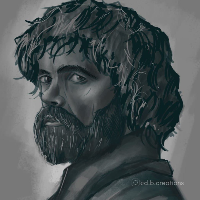
这把我C






举报类型
- 内容涉黄/赌/毒
- 内容侵权/抄袭
- 政治相关
- 涉嫌广告
- 侮辱谩骂
- 其他
详细说明