【深度学习】如何从结构出发更好的改进一个神经网络
【深度学习】如何从结构出发更好的改进一个神经网络
文章目录
1 降采样和升采样
2 UNet++模型诞生
3 参数多了是导致UNet++比UNet好吗
4 一些思路
5 改进卷积结构
5.1 转置卷积
5.2 空洞卷积
5.3 Depth-wise Convolution
5.4 MBConv
5.5 高效的Unet
5.6 基于keras的代码实现
1 降采样和升采样
第一个问题: 降采样对于分割网络到底是不是必须的?问这个问题的原因就是,既然输入和输出都是相同大小的图,为什么要折腾去降采样一下再升采样呢?
理论回答是这样的: 降(下)采样的理论意义,它可以增加对输入图像的一些小扰动的鲁棒性,比如图像平移,旋转等,减少过拟合的风险,降低运算量,和增加感受野的大小。升(上)采样的最大的作用其实就是把抽象的特征再还原解码到原图的尺寸,最终得到分割结果。如下图所示:
浅层结构可以抓取图像的一些简单的特征,比如边界,颜色,而深层结构因为感受野大了,而且经过的卷积操作多了,能抓取到图像的一些说不清道不明的抽象特征,讲的越来越玄学了,总之,浅有浅的侧重,深有深的优势.
多深才好?U-Net为什么只在4层以后才返回去?问题实际是这样的,下图所示,既然 X1,0 、X2,0、 X3,0 、X4,0所抓取的特征都很重要,为什么我非要降到 X4,0 层了才开始上采样回去呢?
2 UNet++模型诞生
这个综合长连接和短连接的方案就是作者他们在MICCAI中发表的UNet++,也就是说这里的短连接是为了使模型能够得到训练,然后长连接是获得更多信息.
(UNet++和刚刚说的那个CVPR的论文结构也太像了吧,这个工作和UC Berkeley的研究是完全两个独立的工作,也算是一个美丽的巧合。UNet++在年初时思路就已经成型了,CVPR那篇是我们七月份开会的时候看到的,当时UNet++已经被录用了,所以相当于同时提出。另外,和CVPR的那篇论文相比,作者还有一个更精彩的点埋在后面说,刚刚也留了一个伏笔)
3 参数多了是导致UNet++比UNet好吗
UNet++的效果是比UNet好,从网络结构上看,说白了就是把原来空心的U-Net填满了
所以有人会认为是参数多了才导致效果好,而不是网络结构的增加.怎么反驳这个呢?
为了回答这个问题,同样作者又去做实验验证
作者的做法是强行增加U-Net里面的参数量,让它变宽,也就是增加它每个层的卷积核个数。由此,作者他们设计了一个叫wide U-Net的参考结构,先来看看UNet++的参数数量是9.04M,而U-Net是7.76M,多了差不多16%的参数,所以wide U-Net我们在设计时就让它的参数比UNet++差不多,并且还稍微多一点点,来证明效果好并不是无脑增加参数量带来的
显然,这个实验用到了控制变量法,为了证明不是参数量影响了模型的表现.所以增加U-Net参数使它变宽,即“wide” U-Net.这样这个“wide” U-Net就和UNet++的参数差不多,甚至还多了点.实验结果如下:
U-Net和FCN为什么成功,因为它相当于给了一个网络的框架,具体用什么特征提取器,随便。这个时候,高引就出现了,各种在encoder上的微创新络绎不绝,最直接的就是用ImageNet里面的明星结构来套嘛,前几年的BottleNeck,Residual,还有去年的DenseNet,就比谁出文章快。这一类的论文就相当于从1到10的递进,而U-Net这个低层结构的提出却是从0到1。说句题外话,像这种从1到10的论文,引用往往不会比从0到1的论文高,因为它不自觉的局限了自己的扩展空间,比如我说,我写一篇论文,说特征提取器就必须是dense block,或者必须是residual block效果好,然后名字也就是DenseUNet或者ResUNet,就这样结束了。所以关于backbone到底用什么的问题,并不是我这次要讲的重点。
4 一些思路
1.改loss,引进新的loss或针对存在的问题魔改loss。2.改架构,引入各种奇奇怪怪的模块,channel attention、spatial attention、pixel attention等等。3.改训练方法or学习方法,lr、batchsize、contrastive learning,都有很多方式可以尝试是否有更好的指标。4.改应用方向。
5 改进卷积结构
5.1 转置卷积
我们将低维特征映射到高维特征的卷积操作称为转置卷积(Transposed Convolution),也称为反卷积.
和卷积网络中,卷积层的前向计算和反向传播也是一种转置关系。
对一个p 维的向量z,和大小为m的卷积核,如果希望通过卷积操作来映射到高维向量,只需要对向量z 进行两端补零p = m− 1,然后进行卷积,可以得到p + m − 1 维的向量。
5.2 空洞卷积
对于一个卷积层,如果希望增加输出单元的感受野,一般可以通过三种方式实现:
(1)增加卷积核的大小;
(2)增加层数;
(3)在卷积之前进行汇聚操作。
空洞卷积(Atrous Convolution),或称为膨胀卷积(Dilated Convolution),是一种不增加参数数量,同时增加输出单元感受野的一种方法。相比原来的正常convolution,dilated convolution 多了一个 hyper-parameter 称之为 dilation rate 指的是kernel的间隔数量(e.g. 正常的 convolution 是 dilatation rate 1)。
Standard Convolution with a 3 x 3 kernel (and padding)
Dilated Convolution with a 3 x 3 kernel and dilation rate 2
空洞卷积通过给卷积核插入“空洞”来变相地增加其大小。如果在卷积核的每两个元素之间插入d − 1 个空洞,卷积核的有效大小为
m′ = m + (m − 1) × (d − 1),
其中d 称为膨胀率(Dilation Rate)。当d = 1 时卷积核为普通的卷积核。
5.3 Depth-wise Convolution
最近看到了一些关于depth-wise 卷积的讨论以及争议,尤其是很多人吐槽EfficientNet利用depth-wise卷积来减少FLOPs但是计算速度却并没有相应的变快。反而拥有更多FLOPs的RegNet号称推理速度是EfficientNet的5倍。非常好奇,这里面发生了什么,为什么计算量小的网络推理速度反而慢于计算量大的网络?
5.4 MBConv
移动翻转瓶颈卷积(mobile inverted bottleneck convolution,MBConv),类似于 MobileNetV2 和 MnasNet,由深度可分离卷积Depthwise Convolution和SENet构成。
每个MBConv的网络结构如下:
MBConv = 1x1升维 + Depthwise Convolution + SENet + 1x1降维 + add
5.5 高效的Unet
以EfficientNet为编码器的Unet的PyTorch 1.0实现
由于解码器路径中存在一些舍入问题(不是错误,因此这是一个功能) 😏),输入形状应可被32整除。
例如224x224是适合输入图像的尺寸,但225x225则不是。
EfficientUnets
例如,说您想要一个带有2个输出通道的预训练的efficiency-b0模型:
具有EfficientNet编码器的Unet
高效网-B0
高效网-B1
EfficientNet-B2
EfficientNet-B3
EfficientNet-B4
高效网-B5
高效网-B6
高效网-B7
5.6 基于keras的代码实现
from keras.layers import *
from keras import models
from .efficientnet import *
from .utils import conv_kernel_initializer
__all__ = ['get_efficient_unet_b0', 'get_efficient_unet_b1', 'get_efficient_unet_b2', 'get_efficient_unet_b3',
'get_efficient_unet_b4', 'get_efficient_unet_b5', 'get_efficient_unet_b6', 'get_efficient_unet_b7',
'get_blocknr_of_skip_candidates']
def get_blocknr_of_skip_candidates(encoder, verbose=False):
"""
Get block numbers of the blocks which will be used for concatenation in the Unet.
:param encoder: the encoder
:param verbose: if set to True, the shape information of all blocks will be printed in the console
:return: a list of block numbers
"""
shapes = []
candidates = []
mbblock_nr = 0
while True:
try:
mbblock = encoder.get_layer('blocks_{}_output_batch_norm'.format(mbblock_nr)).output
shape = int(mbblock.shape[1]), int(mbblock.shape[2])
if shape not in shapes:
shapes.append(shape)
candidates.append(mbblock_nr)
if verbose:
print('blocks_{}_output_shape: {}'.format(mbblock_nr, shape))
mbblock_nr += 1
except ValueError:
break
return candidates
def DoubleConv(filters, kernel_size, initializer='glorot_uniform'):
def layer(x):
x = Conv2D(filters, kernel_size, padding='same', use_bias=False, kernel_initializer=initializer)(x)
x = BatchNormalization()(x)
x = Activation('relu')(x)
x = Conv2D(filters, kernel_size, padding='same', use_bias=False, kernel_initializer=initializer)(x)
x = BatchNormalization()(x)
x = Activation('relu')(x)
return x
return layer
def UpSampling2D_block(filters, kernel_size=(3, 3), upsample_rate=(2, 2), interpolation='bilinear',
initializer='glorot_uniform', skip=None):
def layer(input_tensor):
x = UpSampling2D(size=upsample_rate, interpolation=interpolation)(input_tensor)
if skip is not None:
x = Concatenate()([x, skip])
x = DoubleConv(filters, kernel_size, initializer=initializer)(x)
return x
return layer
def Conv2DTranspose_block(filters, kernel_size=(3, 3), transpose_kernel_size=(2, 2), upsample_rate=(2, 2),
initializer='glorot_uniform', skip=None):
def layer(input_tensor):
x = Conv2DTranspose(filters, transpose_kernel_size, strides=upsample_rate, padding='same')(input_tensor)
if skip is not None:
x = Concatenate()([x, skip])
x = DoubleConv(filters, kernel_size, initializer=initializer)(x)
return x
return layer
# noinspection PyTypeChecker
def _get_efficient_unet(encoder, out_channels=2, block_type='upsampling', concat_input=True):
MBConvBlocks = []
skip_candidates = get_blocknr_of_skip_candidates(encoder)
for mbblock_nr in skip_candidates:
mbblock = encoder.get_layer('blocks_{}_output_batch_norm'.format(mbblock_nr)).output
MBConvBlocks.append(mbblock)
# delete the last block since it won't be used in the process of concatenation
MBConvBlocks.pop()
input_ = encoder.input
head = encoder.get_layer('head_swish').output
blocks = [input_] + MBConvBlocks + [head]
if block_type == 'upsampling':
UpBlock = UpSampling2D_block
else:
UpBlock = Conv2DTranspose_block
o = blocks.pop()
o = UpBlock(512, initializer=conv_kernel_initializer, skip=blocks.pop())(o)
o = UpBlock(256, initializer=conv_kernel_initializer, skip=blocks.pop())(o)
o = UpBlock(128, initializer=conv_kernel_initializer, skip=blocks.pop())(o)
o = UpBlock(64, initializer=conv_kernel_initializer, skip=blocks.pop())(o)
if concat_input:
o = UpBlock(32, initializer=conv_kernel_initializer, skip=blocks.pop())(o)
else:
o = UpBlock(32, initializer=conv_kernel_initializer, skip=None)(o)
o = Conv2D(out_channels, (1, 1), padding='same', kernel_initializer=conv_kernel_initializer)(o)
model = models.Model(encoder.input, o)
return model
def get_efficient_unet_b0(input_shape, out_channels=2, pretrained=False, block_type='transpose', concat_input=True):
"""Get a Unet model with Efficient-B0 encoder
:param input_shape: shape of input (cannot have None element)
:param out_channels: the number of output channels
:param pretrained: True for ImageNet pretrained weights
:param block_type: "upsampling" to use UpSampling layer, otherwise use Conv2DTranspose layer
:param concat_input: if True, input image will be concatenated with the last conv layer
:return: an EfficientUnet_B0 model
"""
encoder = get_efficientnet_b0_encoder(input_shape, pretrained=pretrained)
model = _get_efficient_unet(encoder, out_channels, block_type=block_type, concat_input=concat_input)
return model
该代码的前部分为U-net架构的实现,代码主要功能是修改编码器部分。
在这个部分可以根据你自己的需求,选择不同的EfficientNet基线的改进模型。
就到这啦~~~
卷积层的变体和替代做一个简短的总结
MBConv
在MobileNets: Efficient Convolutional Neural Networks for Mobile Vision Applications 中提出将Conv + BN + ReLU分解为DepthwiseConv + BN +ReLU + PointwiseConv + BN + ReLU.
由深度可分离卷积Depthwise Convolution和SENet构成。
Xception 使用了去掉中间激活函数的变体.
在MobileNetV2中DepthwiseConv被整合到一般的残差结构中用于取代中间的卷积操作, 并去掉了最后一个激活函数. 此外, 与原本先降维再升维相反, 新的残差结构先对输入进行升维.
SE结构在MobileNetV3中被有选择性地加到DepthwiseConv这一层上.
- 分享
- 举报
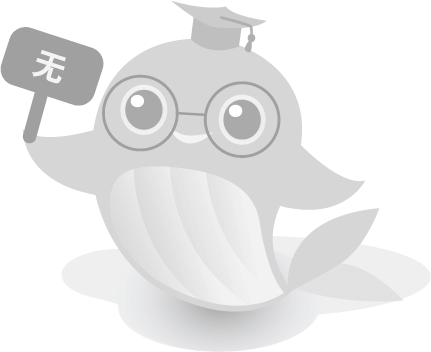
-
浏览量:4011次2021-05-14 09:47:57
-
浏览量:4443次2021-04-23 14:09:15
-
浏览量:4669次2021-04-20 15:50:27
-
浏览量:4000次2018-02-14 10:30:11
-
浏览量:6293次2021-07-14 09:51:09
-
浏览量:5298次2021-08-13 15:39:02
-
浏览量:8456次2021-05-28 16:59:43
-
浏览量:6602次2021-05-31 17:02:05
-
浏览量:4955次2021-04-23 14:09:37
-
浏览量:11757次2021-05-04 20:20:07
-
浏览量:498次2023-08-28 09:56:42
-
浏览量:5488次2021-05-28 16:59:25
-
浏览量:7099次2021-07-26 11:30:25
-
浏览量:499次2024-02-01 14:20:47
-
浏览量:4310次2021-04-19 14:54:23
-
浏览量:16096次2021-06-07 17:47:54
-
浏览量:747次2024-02-01 14:28:23
-
浏览量:10061次2021-06-15 10:30:15
-
浏览量:470次2023-09-28 11:44:09
-
广告/SPAM
-
恶意灌水
-
违规内容
-
文不对题
-
重复发帖
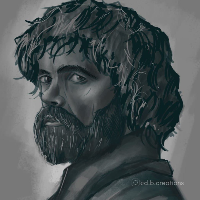
这把我C






举报类型
- 内容涉黄/赌/毒
- 内容侵权/抄袭
- 政治相关
- 涉嫌广告
- 侮辱谩骂
- 其他
详细说明